Technology
👀 Eyes for scooters
6 April, 2022
New article of the fluctuo‘s series: the potential of computer vision in the shared electric mobility industry.
Since the early days of scooter-sharing, back in 2018, the industry has significantly matured to cater to the needs of cities worldwide. Gone are the days of launching in new territories with scooters fit for the consumer market: far superior technology now allows companies to build vehicles that are answering the needs of operators, riders and local authorities simultaneously.
Despite these advances, there are ongoing challenges with scooter sharing and, broadly speaking, with free-floating services. The most pressing issues are parking management and ensuring the safety of all road users. Technology, once again, is coming to the rescue. The ‘smartphone on wheels‘ is getting smarter, and computer vision solutions are being trialed and adopted by major operators.
The timing is perfect for companies developing such solutions: cities are more and more insistent on rider and pedestrian safety, forcing operators to innovate to stand a chance of winning tenders. Computer vision, which basically combines a camera with AI tools to analyze the images, aims to solve those problems and speed up the adoption of shared micromobility.
Tell me where you ride…
With accidents and antisocial behaviours making the headlines in the local news, regulation quickly grew stricter in many cities, especially around parking and sidewalk riding. Scooters were initially located using basic GPS devices, which soon revealed limitations in providing reliable information to both the user – who would look for a vehicle away from its real location – and the operator – who would struggle to monitor the scooter and forward the relevant information.
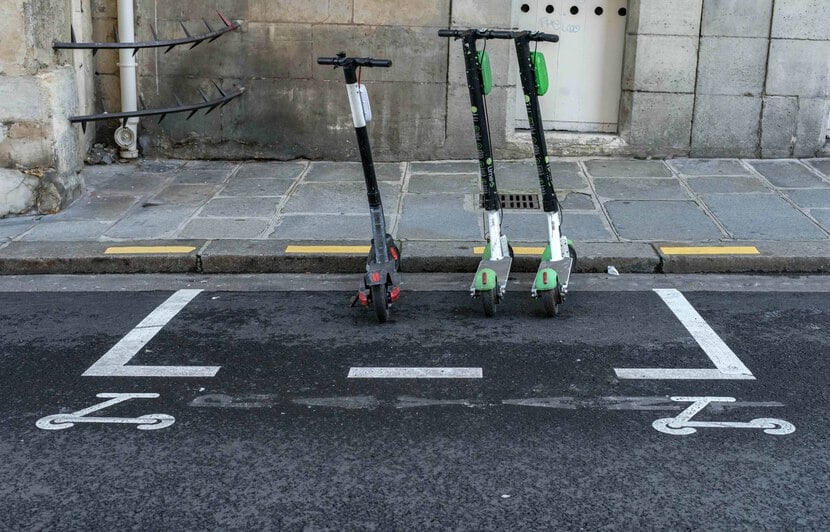
Virtual boundaries (geofencing), defining ‘no-go’, slow or parking zones, quickly became a best practice around the globe to prevent bad riding and parking behaviour… but are just one part of the solution. This is especially true for parking, the accuracy for which is now 10 centimetres, triggering the development of enhanced GPS positioning solutions for many operators convinced of the benefits it could provide to all parties (user, city and themselves).
Different technologies can be employed to improve the GPS signal and provide better accuracy. The most commonly used are:
1/ RTK (real-time kinematic), which compares the phase of positioning satellites signals to correct the actual position
2/ Dual-band GNSS, which takes the average position from two signals, reducing the positioning errors.
3/ Dead reckoning, wherein the case of a lack of GNSS signal, an estimated position is given based on the latest confirmed position and data from multiple sensors (accelerometer, gyroscope…)
Operators, such as Bird or Voi, claim to have reached this 10cm-level accuracy thanks to these technologies. But skeptics have two strong arguments in their hands. First, buildings are creating urban canyons, blocking GNSS signals in many parts of the cities. So if you do not have a signal at all, it is impossible to enhance it. Then, if those technologies allow a cm-level accuracy, it implies that maps with a cm-level accuracy are required, identifying not only the lanes (for pedestrians, cyclists or drivers) but all the street furniture to assess correct parking locations. It doesn’t exist in any city, yet.
To answer the need for hyper-accurate positioning, while overcoming these latest drawbacks, companies have worked on an alternative technology: computer vision.
Replicating human behaviour
Computer vision is based on a different philosophy from accurate positioning. As Alex Nesic, CEO of Drover AI explains: “Humans are not working with GPS coordinates to communicate about their exact location: they look at their direct environment. We are trying to get closer to that”. With computer vision, you do not need to have a super-accurate positioning: if you know that a scooter is in a 10m radius, you just want to be sure that it is being used and parked in the appropriate places.
With a computer vision solution, an algorithm analyzes images from a camera built-in to an IoT device to understand its environment. The two main companies currently testing the solution in the shared mobility industry, Luna from Dublin (IE) and Drover AI from Los Angeles (US), are using slightly different methods: a forward-facing camera, and an algorithm based on line segmentation for Luna, a ground-facing camera with an algorithm based on object detection for Drover AI.
Both companies currently have two main goals to limit conflicts and accidents with pedestrians. Lane detection, from pavement to cycle lane and road, will prevent pavement riding, while parking identification will ensure the public right of way, and enforce compliance with local regulation (mandatory parking zones). After “training” in local streets, the algorithm is able to adapt to any city and understand its road infrastructure specificities.
Given how recently computer vision has been adopted by the shared mobility industry, devices have been developed externally, and currently are add-on devices that fit on the steering tube of scooters. As external devices, they have been developed to be self-sufficient with just a power source connection. “The redundancy between the scooter IoT and our computer vision devices are important, from GSM to GPS modules. We are currently working on the integration with OEM to drastically reduce the costs of our solution”, explains Alex Nesic. Same strategy for Luna: “Our goal is to lower the cost by around a third to reach $80 per vehicle in 12 to 18 months” according to Ronan Furlong, Luna’s CBO.
Benefits for all stakeholders
Both companies have signed partnerships with major operators to test their solutions in real operating conditions. Luna is working with Voi in the UK and the Nordics, and with Tier in Dublin, while Drover AI mostly worked with Spin (acquired by Tier) and Helbiz in the USA, and recently announced new pilots with Bolt in Oslo, and Beam in Australia. Tier, through its recent acquisition of Fantasmo (who offers a parking control solution using the camera of the user’s smartphone), is actually working on 3 different computer vision solutions. Operators are betting on a solution that is proving their will to work on safety for both the rider and street users, a must to remain competitive in tenders and RFP procedures around the globe. A win-win partnership with companies such as Luna and Drover AI who also need financial support to develop their solutions.
Both companies have signed partnerships with major operators to test their solutions in real operating conditions. Luna is working with Voi in the UK and the Nordics, and with Tier in Dublin, while Drover AI mostly worked with Spin (acquired by Tier) and Helbiz in the USA, and recently announced new pilots with Bolt in Oslo, and Beam in Australia. Tier, through its recent acquisition of Fantasmo (who offers a parking control solution using the camera of the user’s smartphone), is actually working on 3 different computer vision solutions. Operators are betting on a solution that is proving their will to work on safety for both the rider and street users, a must to remain competitive in tenders and RFP procedures around the globe. A win-win partnership with companies such as Luna and Drover AI who also need financial support to develop their solutions.
If all operators are interested in lane detection and good parking control features, they also realise the potential of computer vision for further improvements in their operations. Depending on the local specificities, operators are asking for additional developments: “We are working on multiple new projects, from detecting blocked footpaths to tipped over scooters or black ice. Our vision is to offer an app-store product where operators will be able to pick the features they want”, develops Ronan Furlong. On top of it, Drover AI and Luna both confirm working on electric bicycles and considering equipping mopeds in the future, a big plus for operators with multimodal fleets.
The benefits of computer vision for shared mobility services are even reaching the transport authorities of major cities who are calling companies to understand more about the technology and assess how mature the products are. Some comforting messages in favour of computer vision solutions are coming from American cities: the Chicago Department of Transportation released a scooter sharing RFP asking applicants to “describe the sidewalk riding detection hardware and software they will deploy” in the city. Spin was also able to enter the Seattle market after the licenses were awarded, as the city was willing to test Drover AI’s solution. On top of operators’ consideration about safety and parking compliance, cities might also think about the data they could get from the vehicles equipped with computer vision devices to feed their decisions in urban planning.
It’s early days for computer vision, but everything points towards successful applications that bring plenty of benefits for all shared mobility stakeholders: operational efficiency for operators, enforcement of local regulation for operators and cities, urban planning data for cities, safer and smoother rides for users. If (when?) scalability is proven, the development potential for light electric vehicles manufacturers (to improve safety, lower insurance costs…) or operators of other shared vehicles (e-bikes, mopeds) with their own constraints are huge.
Written by Alexandre Gauquelin (Shared Micromobility) and Julien Chamussy (fluctuo)
Video ressources
Intro Drover AI : https://youtu.be/2KpWGfDytTw
Luna sidewalk detection : https://youtu.be/6JEth9sK14Q
Luna sidewalk detection Stockholm : https://youtu.be/mn98aTBFb7w